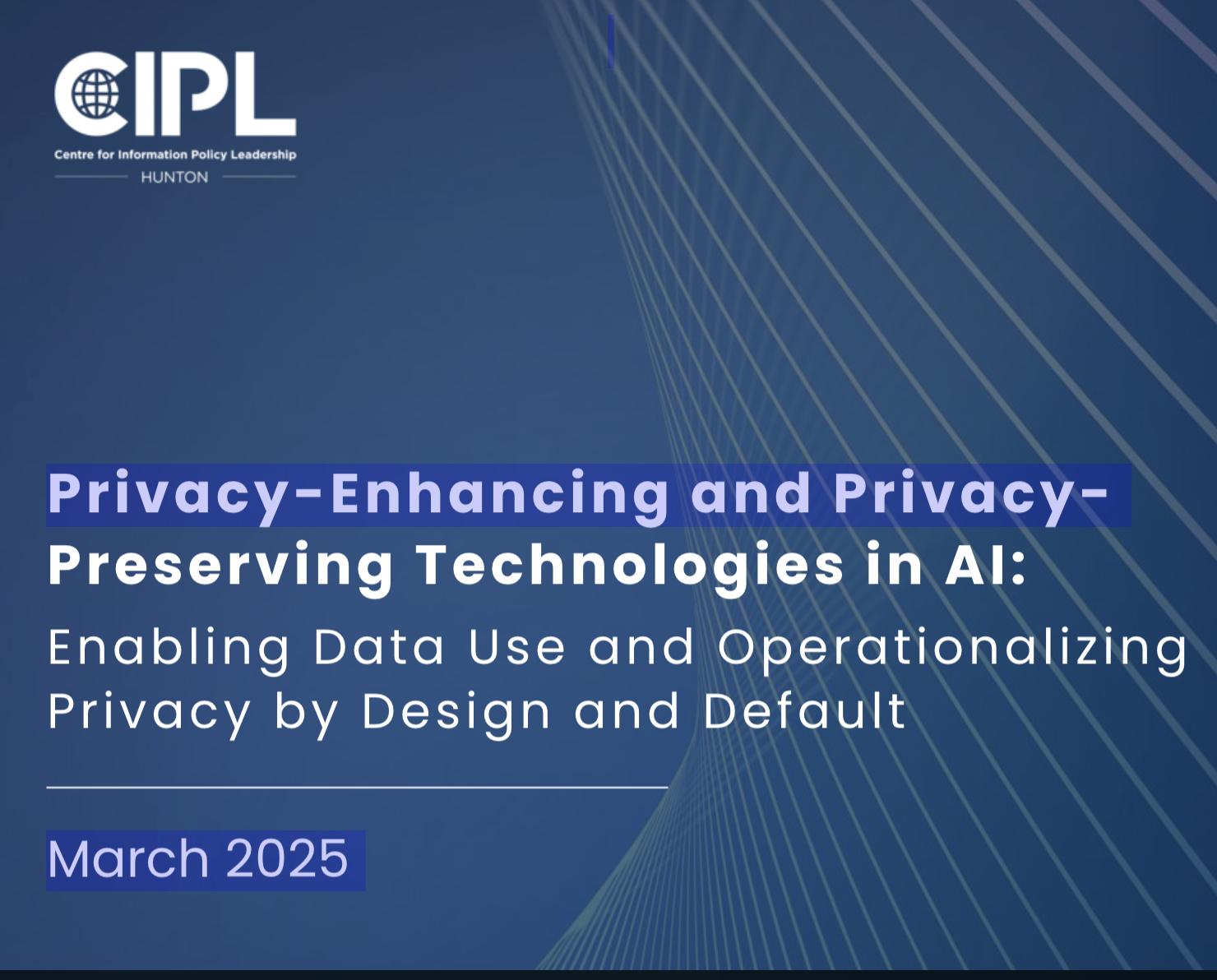
:
🔐 Empowering responsible AI: How privacy-enhancing technologies are shaping the future of data sharing
The report titled "Privacy-enhancing technologies in AI: enabling data use and operationalizing privacy by design and default" (March 2025), published by the Centre for Information Policy Leadership (CIPL), explores how Privacy-Enhancing Technologies (PETs) can support the responsible development and deployment of AI systems. It provides a deep dive into how various PETs mitigate privacy risks, protect sensitive data, and promote data utility across the AI lifecycle—from model training to deployment.
Key Highlights
1. Why PETs matter in AI
As AI increasingly relies on vast datasets—including personal and sensitive information—privacy, security, and compliance challenges mount. PETs offer a crucial solution by enabling data sharing and use without compromising individual rights or commercial interests. CIPL frames PETs as not just privacy tools but business enablers that promote trust, regulatory compliance, and innovation.
2. Types of PETs and their applications
a) Synthetic Data
Artificially generated to mirror real data, synthetic data enables model training and testing without using actual personal data. It’s particularly valuable in medical research and AI development where privacy is critical.
Examples:
-
InstructLab project using synthetic data to fine-tune large language models.
-
AI palm recognition systems trained on synthetic hand images to reduce data collection needs.
b) Homomorphic Encryption (HE)
Allows computations on encrypted data, preserving confidentiality throughout model training, validation, and deployment. Often paired with federated learning.
Examples:
-
Used with federated learning to enhance privacy in cross-organization collaboration.
-
Applied in photo tagging systems to allow private search functionalities.
c) Differential Privacy (DP)
Adds noise to data to prevent identification of individuals. It’s widely used in model training and deployment to protect against data inference attacks.
Examples:
-
Used in photo libraries and AI safety classifiers to ensure user privacy.
-
Enables generation of differentially private synthetic text and training data.
d) Federated Learning and Analysis
Trains AI models across multiple decentralized datasets without sharing raw data. Effective in contexts with legal or logistical constraints on data transfer.
Examples:
-
Cancer detection models trained across hospitals using federated learning and Trusted Execution Environments (TEEs).
-
Fraud detection in insurance using federated learning and synthetic data.
e) Trusted Execution Environments (TEEs)
Secure hardware zones that allow safe execution of code and storage of sensitive data. Used in cloud-based generative AI and on-device processing.
Examples:
-
Used to train models on client financial data without exposing raw inputs.
-
Applied to process encrypted prompts in AI systems.
f) Secure Multi-Party Computation (SMPC)
Allows multiple parties to compute jointly on private data without revealing it. Useful for collaboration where privacy is paramount.
Examples:
-
Neuroimaging studies involving rs-fMRI data conducted via SMPC to preserve patient confidentiality.
3. Balancing privacy and utility
The report emphasizes that privacy protection must not diminish data utility. Organizations are advised to adopt a nuanced approach—combining PETs and adapting noise levels or cryptographic techniques depending on context.
4. PETs Beyond Privacy
PETs:
-
Improve data quality (e.g., reduce bias using synthetic data),
-
Enable secure collaboration (e.g., cross-sector AI development),
-
Enhance trust (e.g., compliance with global regulations),
-
Support personalization (e.g., safer on-device AI),
-
Strengthen security (e.g., cryptographic protections).
5. Challenges and Recommendations
Technical and Legal Obstacles
-
High computational demands (especially in homomorphic encryption).
-
Lack of standardized definitions and interoperability.
-
Regulatory uncertainty around what constitutes anonymization.
Recommendations
-
Regulators should provide clearer, practical guidance and adopt a risk-based approach to anonymization.
-
Deployers must ensure transparency and uphold user rights, even when data is obscured.
-
Policymakers and Industry should invest in compute infrastructure and regulatory sandboxes for experimentation.
-
Stakeholders should take a holistic view of PETs—recognizing their strategic and compliance value, not just privacy protection.
Conclusion
The report reinforces that PETs are foundational to operationalizing “privacy by design and default” in AI. While not a panacea, their thoughtful integration into AI systems can drive innovation, trust, and accountability across sectors.
📘 For a deeper dive into case studies, technical insights, and policy guidance, read the full report by the Centre for Information Policy Leadership (CIPL):
Privacy-Enhancing Technologies in AI: Enabling Data Use and Operationalizing Privacy by Design and Default (March 2025).